A Picture is Worth a Thousand Labels: Best Practices for Image Data Collection in ML
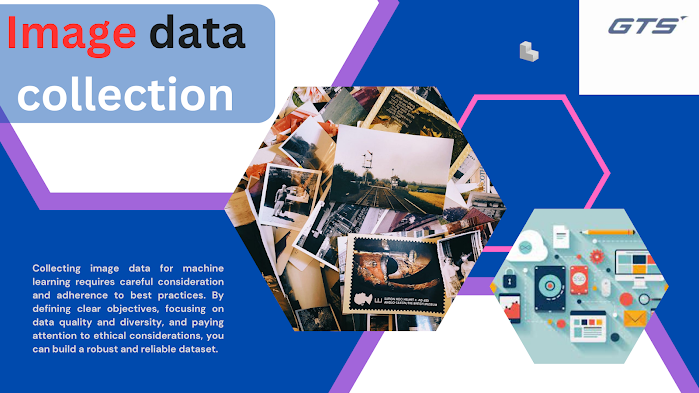
Introduction:
In the field of machine learning, image data plays a crucial role in training models for various applications such as object recognition, image classification, and image generation. The accuracy and reliability of these models heavily depend on the quality and diversity of the image data used for training. Collecting image data can be a complex and time-consuming task, but following best practices can greatly enhance the effectiveness of the training process. In this blog post, we will discuss some key considerations and best practices for Image data collection in machine learning.
Collecting high-quality and diverse image data is essential for training effective machine learning models. The success of these models heavily relies on the quality and representation of the images used during the training process. In this blog post, we will explore best practices for image data collection in machine learning, ensuring that the collected data sets the foundation for accurate and robust model
Define Clear Objectives
Before embarking on the image data collection process, it is crucial to define clear objectives for your machine learning project. Determine the specific tasks and goals that your model needs to achieve. This clarity will guide your data collection efforts and help you focus on acquiring relevant images. Whether it is object recognition, image classification, or image generation, understanding your objectives will enable you to gather the most suitable images for your specific use case.
Ensure Data Quality and Diversity
Data quality and diversity are key factors in building effective machine learning models. When collecting image data, it is important to prioritize quality over quantity. Ensure that the images are of high resolution, properly lit, and visually clear. Removing irrelevant or low-quality images from your dataset will prevent noise and improve model performance.
.png)
Additionally, diversity plays a crucial role in training models that can generalize well to new and unseen data. Collect images that encompass a wide range of variations, including different angles, lighting conditions, backgrounds, and object poses. Incorporating images from various sources and perspectives helps mitigate bias and ensures that your model can handle real-world scenarios more effectively.
Conclusion:
Collecting image data for machine learning requires careful consideration and adherence to best practices. By defining clear objectives, focusing on data quality and diversity, and paying attention to ethical considerations, you can build a robust and reliable dataset. Remember that the success of your machine learning models heavily depends on the quality and representation of the images used for training. By following these best practices, you will be well-equipped to gather the necessary image data to develop accurate and effective machine learning models. Regenerate response
Comments
Post a Comment