Data is King: Exploring the Importance of Data Collection for Machine Learning
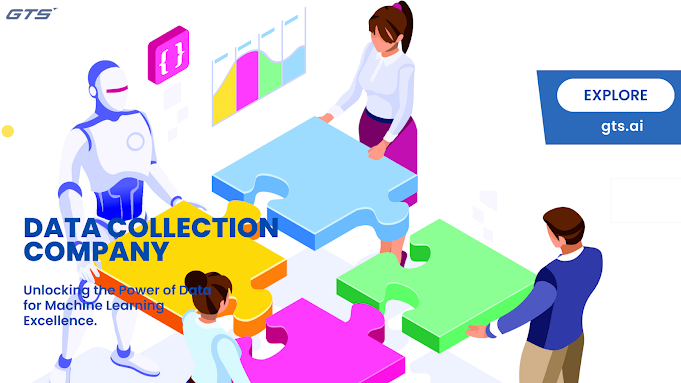
Introduction:
In the realm of machine learning, data holds paramount importance. The success and effectiveness of machine learning algorithms heavily rely on the quality, quantity, and representativeness of the data used for training. This article delves into the crucial role of data collection in machine learning, highlighting its significance in building accurate models, addressing common challenges in Data collection company , and exploring strategies for acquiring high-quality datasets.
The Foundation of Machine Learning: Quality Data Collection
Quality data is the bedrock upon which successful machine learning models are built. This section emphasizes the importance of collecting clean, reliable, and diverse datasets. It discusses the significance of data labeling and annotation, ensuring data integrity through rigorous quality control measures, and handling biases and imbalances within the collected data. Furthermore, it explores techniques such as data augmentation and synthetic data generation to enhance dataset diversity and mitigate the risk of overfitting. Additionally, it delves into the significance of data provenance, documentation, and metadata to ensure transparency and reproducibility in machine learning experiments
Overcoming Challenges in Data Collection
Data collection for machine learning poses several challenges that need to be addressed to ensure the reliability and validity of the collected datasets. This section explores common challenges such as data privacy and ethical considerations, data accessibility and availability, and the need for large-scale datasets to capture complex patterns. It discusses strategies for navigating these challenges, including leveraging privacy-preserving techniques, establishing data-sharing agreements and collaborations, and exploring alternative sources such as open data repositories and data marketplaces. Moreover, it examines the role of data governance frameworks and regulatory compliance in ensuring responsible and ethical data collection practices.
.png)
Conclusion:
Data collection is the foundation upon which successful machine learning models are built. The quality, quantity, and representativeness of the collected data directly impact the accuracy and generalizability of machine learning algorithms. By emphasising the importance of data labelling, quality control, diversity, and transparency, organisations can acquire high-quality datasets that drive the development of accurate and robust machine learning models.
Additionally, by addressing challenges such as data privacy, accessibility, and scalability, stakeholders can foster responsible and ethical data collection practices. As machine learning continues to advance, recognizing the significance of data collection and investing resources in acquiring and curating high-quality datasets will be instrumental in unlocking the true potential of machine learning applications across various domains.
How GTS.AIcan be a right data collection company
GTS.AI can be a right data collection company for several reasons. First, GTS.AI is an experienced and reputable company with a proven track record of providing high-quality Image data collection services to a diverse range of clients. They have a team of skilled professionals who are knowledgeable in various data collection techniques and technologies, allowing them to deliver customized solutions to meet the unique needs of each client.
Comments
Post a Comment