Precision Mapping: Exploring ML Annotation in ADAS Training
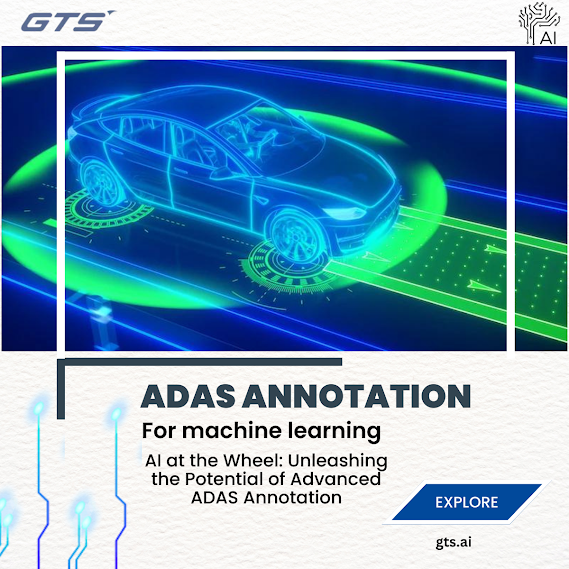
Introduction:
Advanced Driver Assistance Systems (ADAS) rely on machine learning algorithms to enhance vehicle safety and improve driving experiences. One critical component of ADAS training is the annotation of data to enable the machine learning models to accurately detect and respond to various objects and events on the road.
This article delves into the significance of ML annotation in ADAS training, highlighting its role in building robust models, addressing challenges in annotation, and discussing strategies for achieving precise mapping of objects and events in ADAS Annotation datasets.
The Role of ML Annotation in ADAS Training
ML annotation plays a crucial role in ADAS training by providing labeled data that enables machine learning models to recognize and interpret objects and events on the road. This section explores the significance of accurately annotating objects such as pedestrians, vehicles, traffic signs, and road boundaries.
It discusses annotation techniques, including bounding boxes, semantic segmentation, and instance segmentation, to precisely identify and delineate objects of interest.
Furthermore, it highlights the importance of temporal annotation to capture dynamic events, such as lane changes and traffic flow. Accurate and comprehensive annotation serves as the foundation for training ADAS models that can make informed decisions and respond effectively to real-world driving scenarios.

Challenges and Strategies in ML Annotation for ADAS Training
ML annotation for ADAS training presents unique challenges that require careful consideration and effective strategies. This section discusses common challenges, including the complexities of annotating occluded objects, handling variations in lighting conditions, and ensuring consistent annotation across different annotators.
It explores strategies for addressing these challenges, such as employing advanced annotation tools with intelligent assistance, leveraging synthetic data and simulation techniques to augment real-world datasets, and establishing robust quality control measures to ensure annotation accuracy and consistency.
Additionally, it highlights the importance of continuous feedback loops and iterative refinement in the annotation process to improve the quality and reliability of ADAS data collection training datasets.
Conclusion:
ML annotation is a critical aspect of ADAS training, enabling machine learning models to accurately detect and respond to objects and events on the road. Precise mapping of objects and events through accurate annotation serves as the foundation for building robust ADAS models that enhance vehicle safety and driving experiences. By emphasizing the role of ML annotation, organizations can address challenges such as occlusions, lighting variations, and consistency in annotation. Implementing effective annotation strategies, leveraging advanced tools, and incorporating iterative refinement processes will result in high-quality and reliable ADAS training datasets.
.png)
GTS.AI offers best services of the Adas Annotation
GTS.AI may offer ADAS Annotation services to companies developing ADAS systems. These services may include manual annotation by trained professionals, as well as automated annotation using machine learning algorithms. The quality and accuracy of these services may vary depending on a number of factors, including the expertise of the annotators, the quality of the data being annotated, and the specific requirements of the project. GTS) is an AI Data Collection Company that provides different Datasets like image datasets, video datasets, text datasets, speech datasets, etc. to train your machine learning model.
Comments
Post a Comment