The Road to Intelligent Vehicles: Leveraging ADAS Data for ML Success
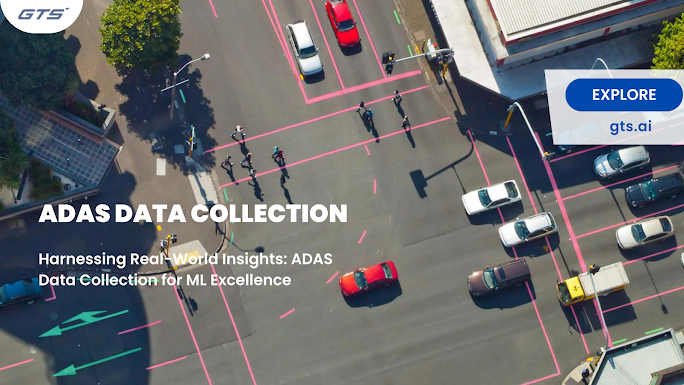
Introduction:
The automotive industry has been rapidly evolving, with one of the most significant advancements being the integration of advanced driver assistance systems (ADAS) into vehicles. ADAS technologies, such as lane-keeping assist, adaptive cruise control, and automatic emergency braking, have made driving safer and more convenient. However, the real potential of ADAS lies in its ability to generate massive amounts of valuable data that can be harnessed to train machine learning (ML) algorithms. In this blog, we will explore the road to intelligent vehicles and discuss how leveraging ADAS data collection can lead to ML success.
What is ADAS?
ADAS refers to a collection of technologies designed to assist drivers and automate various aspects of the driving process. These systems utilize a combination of sensors, cameras, radar, and other data sources to monitor the vehicle's surroundings and provide warnings or take actions to prevent collisions or accidents. Some common examples of ADAS features include adaptive cruise control, lane-keeping assist, automatic emergency braking, and blind-spot monitoring.
The Power of ADAS Data
ADAS systems generate a wealth of valuable data about the vehicle's environment, including road conditions, traffic patterns, pedestrian movement, and other vehicles' behavior. This data, when collected and analyzed effectively, can serve as a goldmine for training and refining ML models for autonomous driving.
- Training and Validation: ADAS data can be used to train ML algorithms to recognize and understand various objects and scenarios on the road. By leveraging real-world data captured by ADAS sensors, ML models can learn to identify lane markings, traffic signs, pedestrians, and other vehicles with a high degree of accuracy. This data-driven approach enables the development of robust and reliable ML models that can adapt to different driving conditions.
- Simulation and Testing: ADAS data can be used to create virtual simulations for testing and validating autonomous driving algorithms. By recreating real-world driving scenarios using ADAS data, ML models can be subjected to a wide range of complex situations without the need for physical prototyping. This approach accelerates the development cycle, reduces costs, and ensures that autonomous vehicles are thoroughly tested before being deployed on public roads.
- Anomaly Detection: ADAS data can also be utilized to detect and analyze anomalies or unusual events on the road. ML algorithms can be trained to identify abnormal behavior, such as sudden braking, swerving, or unexpected obstacles. This capability enhances safety by alerting drivers or triggering automated responses to mitigate potential risks.

Challenges and Considerations
While ADAS data holds tremendous potential, there are several challenges and considerations that need to be addressed to leverage it effectively for ML success:
- Data Quality and Quantity: ADAS data should be of high quality, accurately capturing real-world driving scenarios. The quantity of data is also crucial, as ML algorithms require large amounts of diverse and representative data to learn effectively.
- Data Privacy and Security: ADAS data often contains sensitive information, such as location data and driving behavior. Safeguarding this data and ensuring privacy is of utmost importance to gain public trust in autonomous driving technology.
- Data Standardization: ADAS systems are implemented by various automotive manufacturers, resulting in differences in data formats and protocols. Standardizing ADAS data can facilitate interoperability and collaboration among different stakeholders in the autonomous driving ecosystem.
Conclusion
The road to intelligent vehicles heavily relies on leveraging ADAS data for ML success. The vast amounts of data generated by ADAS annotation systems can be harnessed to train ML algorithms, validate autonomous driving models through simulations, and enhance anomaly detection capabilities. By addressing the challenges of data quality, privacy, and standardization, we can unlock the full potential of ADAS data and accelerate the development of safe and reliable autonomous driving systems.
ADAS Data Collection and GTS
Did you ever imagine that your family automobile might be equipped with RADAR and SONAR as aero planes and submarines do? Did you even know what LiDAR stood for? Did you picture a dashboard with flat-screen displays and a navigation system linked to satellites in space? It would have appeared to be science fiction and would have been out of reach for at least 100 years. But all of that and more is now a reality. ADAS addresses the most critical aspect of travel: human safety. Because human mistake causes more than 90% of road accidents, injuries, and fatalities, every breakthrough in ADAS has a clear and absolute effect on preventing injuries and deaths. Data Collection Company provides ADAS Dataset Collection, Image Data Collection services to train, test and validate your models. We provide ADAS Datasets Collection services in different regions and geographies like the USA, India, Germany, Europe, etc. Our name has been recognised by big brands, and we never compromise on our services.
Comments
Post a Comment