Boosting Performance: Leveraging High-Quality ADAS Annotation in AI Machine Learning
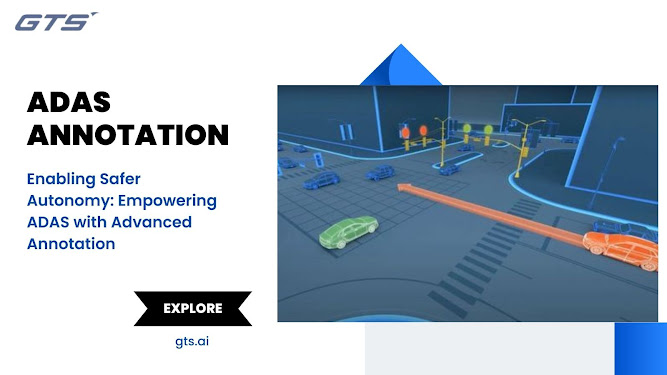
Introduction:
Advanced Driver Assistance Systems (ADAS) are revolutionising the automotive industry, enhancing driver safety, and paving the way for autonomous vehicles. AI machine learning models power these systems, enabling them to detect and understand the surrounding environment accurately. However, the performance and reliability of ADAS models heavily rely on high-quality ADAS annotation. This blog explores the significance of ADAS annotation in AI machine learning and highlights how leveraging high-quality annotations can boost performance and ensure safer driving experiences.
The Importance of ADAS Annotation in AI Machine Learning:
ADAS annotation plays a crucial role in training AI machine learning models for autonomous driving. Here's why it is essential:
- Training Object Detection and Recognition Models: ADAS annotation involves labelling objects and elements in the driving environment, such as pedestrians, vehicles, traffic signs, and road markings. These annotations serve as ground truth for training object detection and recognition models. By leveraging high-quality ADAS annotation, models can accurately identify and classify objects, enabling robust decision-making and precise response in real-time driving scenarios.
- Ensuring Safety and Reliability: Accurate ADAS annotation is vital for ensuring safety and reliability in autonomous driving systems. Annotated data helps models understand the spatial relationships between different objects, predict their movements, and make informed decisions. Precise annotations enable ADAS models to detect potential hazards, anticipate risks, and take appropriate actions, ultimately enhancing the safety of passengers, pedestrians, and other vehicles on the road.
- Handling Complex Driving Scenarios: ADAS annotation enables models to handle complex driving scenarios, including crowded urban environments, highway driving, and adverse weather conditions. Annotations provide contextual information about the driving environment, such as lane boundaries, traffic signs, and traffic flow. By training on diverse and accurately annotated data, such as Image data, Video data collection, text data.models become more robust and adaptable, ensuring optimal performance in a wide range of challenging situations.

Leveraging High-Quality ADAS Annotation for Performance Boost:
- Expert Annotation Teams: Collaborate with expert annotation teams experienced in ADAS annotation. These teams possess domain knowledge and expertise in accurately labelling objects, understanding driving scenarios, and following annotation guidelines. Their expertise ensures high-quality annotations and reduces potential errors or inconsistencies.
- Consistent Annotation Guidelines: Establish consistent annotation guidelines to ensure uniformity and accuracy across the annotated data. Clear guidelines help annotation teams understand the desired labelling conventions, handle ambiguous cases, and maintain consistency throughout the annotation process. Regular communication and feedback loops between the annotation teams and the company are crucial for refining and improving annotation guidelines.
- Multiple Annotation Perspectives: Incorporate multiple annotation perspectives to capture different viewpoints and enhance model robustness. This includes annotations from different sensors, such as cameras, LiDAR, and radar, providing a comprehensive understanding of the driving environment. Combining multiple perspectives improves object detection, depth perception, and overall situational awareness for ADAS models.
- Continuous Data Quality Assessment: Regularly assess the quality of the annotated ADAS data to identify and address any issues or inconsistencies. Implement rigorous quality control measures, including manual checks, automated verification, and inter-annotator agreement evaluations. Continuous data quality assessment ensures the reliability and accuracy of the annotated data and enhances the performance of ADAS models.

Conclusion:
High-quality ADAS annotation is crucial for training AI machine learning models that power advanced driver assistance systems. Accurate and precise annotations enable models to detect and understand the driving environment, ensuring safety, reliability, and optimal performance. By leveraging expert annotation teams, consistent guidelines, multiple perspectives, and continuous data quality assessment, companies can unlock the full potential of ADAS models, contributing to safer and more efficient autonomous driving experiences.
Comments
Post a Comment