Driving ML Innovation: Key Considerations for ADAS Data Collection
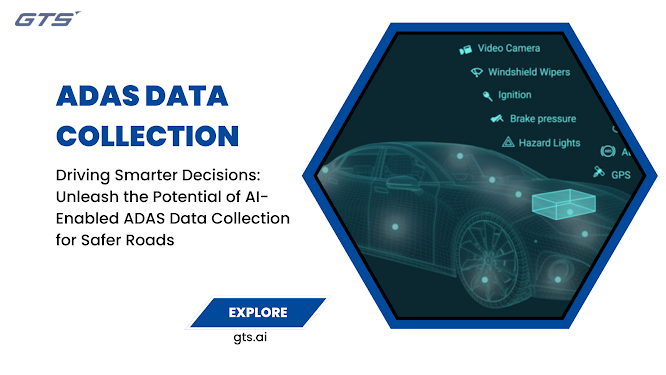
Introduction:
The rapid advancement of advanced driver assistance systems (ADAS) has transformed the automotive industry, paving the way for safer and more autonomous driving experiences. Central to the development of robust ADAS technologies is the collection of high-quality ADAS data. This data serves as the fuel for training machine learning (ML) algorithms, enabling them to make accurate and informed decisions in real-time driving scenarios. In this blog post, we will explore the key considerations for ADAS data collection, highlighting its significance in driving ML innovation for ADAS technologies.
The Importance of ADAS Data Collection:
ADAS data collection plays a critical role in training ML models that power ADAS technologies. By capturing real-world driving scenarios and sensor data, ADAS data provides the necessary inputs for ML algorithms to learn and understand complex driving environments. Here's why ADAS data collection is crucial for ML innovation in ADAS technologies:
- Training ML Models: ADAS data serves as the training dataset for ML algorithms that underpin ADAS technologies. By collecting a diverse range of data, including sensor readings, images, and other relevant information, ML models can learn to identify objects, interpret road conditions, and make accurate predictions in real-time.
- Realistic Driving Scenarios: ADAS data collection involves capturing data from real-world driving scenarios, encompassing various weather conditions, traffic patterns, and road types. This realistic data provides ML models with exposure to different driving situations, enhancing their ability to generalise and perform effectively in diverse environments.
- Sensor Fusion: ADAS systems rely on data from multiple sensors, such as cameras, LiDAR, radar, and GPS, to perceive the driving environment accurately. Collecting synchronised data from these sensors allows ML algorithms to fuse and integrate information, enabling comprehensive perception and precise decision-making.
- Anomaly Detection: Data collection company facilitates the identification and annotation of anomalous events or situations on the road. This annotated data helps ML models learn to recognize and respond appropriately to abnormal driving conditions, such as sudden obstacles, road hazards, or unusual traffic patterns.
- Safety and Risk Analysis: ADAS data collection enables the analysis of driving patterns, identifying potential safety risks and areas for improvement. By examining driver behaviour, traffic situations, and accident data, ML models can be trained to detect risky driving behaviours and provide timely warnings or interventions.
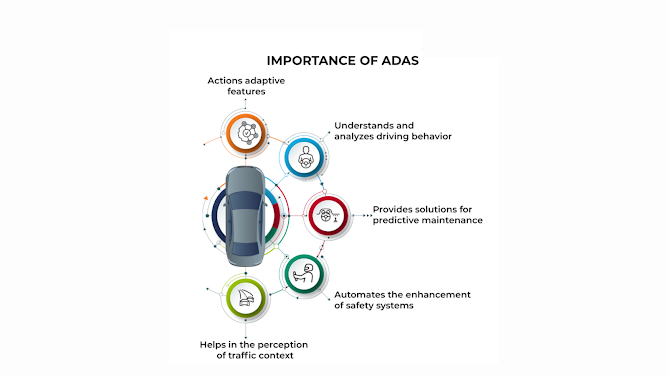.png)
Key Considerations for ADAS Data Collection:
To ensure the effectiveness and relevance of ADAS data collection, several key considerations should be taken into account:
- Data Diversity: Collect ADAS data from a wide range of driving scenarios, encompassing urban, rural, highway, and adverse weather conditions. Include variations in traffic density, road infrastructure, and driving patterns to create a diverse and representative dataset.
- Sensor Calibration: Ensure accurate calibration and synchronisation of sensors to capture precise and aligned data. This calibration is essential for accurate perception and sensor fusion, improving the reliability of ML models.
- Data Annotation: Annotate ADAS data with ground truth labels, including object detection, lane markings, road signs, and other relevant information. This annotated data serves as the reference for training ML models, providing them with the necessary supervision to learn and make accurate predictions.
- Data Privacy and Ethics: Respect data privacy and comply with ethical guidelines when collecting ADAS data. Anonymize personal information, protect user privacy, and adhere to data protection regulations to ensure responsible data collection practices.
- Scalability and Storage: Design a scalable data collection infrastructure that can handle large volumes of ADAS data. Implement efficient storage solutions and data management strategies to accommodate the growing dataset size and enable easy retrieval for ML training.
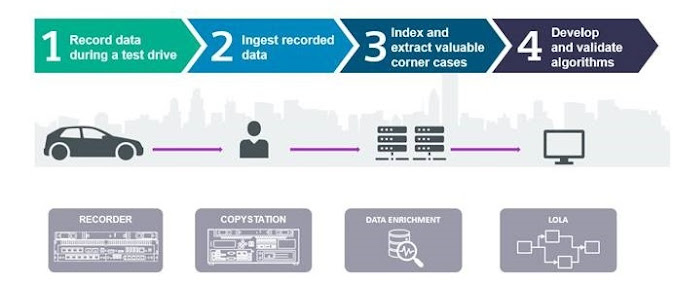
Conclusion:
ADAS data collection is a fundamental component of driving ML innovation in ADAS technologies. By capturing diverse and representative real-world driving scenarios, ADAS data provides ML algorithms with the necessary inputs to learn, adapt, and make accurate decisions. Key considerations such as data diversity, sensor calibration, annotation, privacy, and scalability play a crucial role in ensuring the effectiveness and relevance of ADAS data collection. By prioritising these considerations, companies can propel ML innovation, advancing the capabilities of ADAS technologies and ultimately enhancing road safety and driving experiences.
ADAS Data Collection and GTS
Did you ever imagine that your family automobile might be equipped with RADAR and SONAR as aero planes and submarines do? Did you even know what LiDAR stood for? Did you picture a dashboard with flat-screen displays and a navigation system linked to satellites in space? It would have appeared to be science fiction and would have been out of reach for at least 100 years. But all of that and more is now a reality. ADAS addresses the most critical aspect of travel: human safety. Because human mistake causes more than 90% of road accidents, injuries, and fatalities, every breakthrough in ADAS Annotation has a clear and absolute effect on preventing injuries and deaths.
Comments
Post a Comment