Optimising Video Data Collection for Machine Learning: Key Considerations
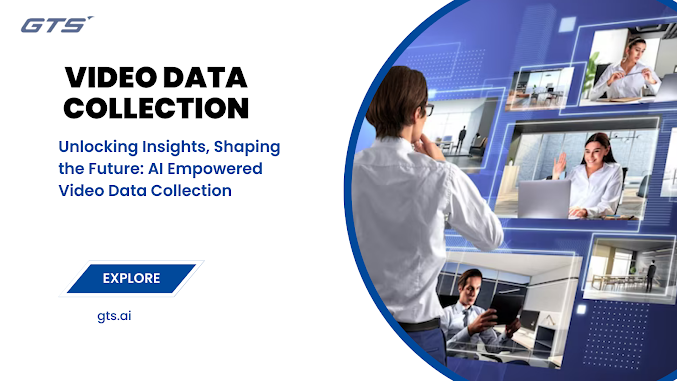
Introduction:
Video data collection has become an invaluable resource for training machine learning (ML) models, enabling a wide range of applications in fields such as computer vision, object detection, activity recognition, and more. However, collecting high-quality video data that aligns with specific ML objectives requires careful planning and consideration. In this blog post, we will explore the key considerations for optimising video data collection to enhance the performance and effectiveness of ML models.
Define the Objective:
Before initiating video data collection, it is crucial to clearly define the ML objective. Determine the specific tasks or challenges the ML model needs to address, such as object recognition, action classification, or scene understanding. This clarity will guide the selection of relevant video content, annotation requirements, and evaluation metrics.
Select a Diverse Range of Content:
To develop robust and generalizable ML models, it is essential to collect a diverse range of video content. This includes variations in lighting conditions, camera angles capture Image data collection backgrounds, and object appearances. Incorporating different scenes, settings, and demographics ensures that the ML model can handle real-world scenarios and generalise well to unseen data.
Consider Annotation Requirements:
Decide on the level of annotation needed for the ML task. Annotations can include bounding boxes, object labels, temporal segmentation, key points, or semantic segmentation masks. Balancing the level of annotation complexity with the available resources is essential. Automated annotation techniques, such as using pre-trained models or crowdsourcing platforms, can help scale the annotation process effectively.
.png)
Ensure Data Quality:
Maintaining high-quality video data is crucial for training reliable ML models. Pay attention to factors such as resolution, frame rate, compression artefacts, and audio quality. Minimise noise, distortion, or motion blur that could hinder model performance. Regularly assess the data quality and implement quality control measures during the collection process.
Capture Varied Perspectives and Actions:
To enhance the model's ability to handle different viewpoints and actions, capture video data from various perspectives and angles. This can involve using multiple cameras, changing camera positions, or utilising different recording devices. Ensure the dataset includes a broad range of activities, gestures, and movements relevant to the ML task.
Consider Ethical and Privacy Considerations:
Respect ethical and privacy considerations when collecting video data. Obtain proper consent and adhere to legal requirements. Anonymize or blur identifiable information to protect individuals' privacy. Establish clear guidelines and processes to handle sensitive or confidential content appropriately.
Data Augmentation and Synthesis:
To expand the size and diversity of the video dataset, consider data augmentation and synthesis techniques. These methods can artificially create new training examples by applying transformations such as cropping, flipping, rotation, or adding realistic noise. Data augmentation helps to improve the model's robustness, generalisation, and performance under different conditions.
Establish Ground Truth and Evaluation Metrics:
Establish ground truth annotations for a subset of the video data to serve as a reference for model evaluation. Determine appropriate evaluation metrics that align with the ML objective. These metrics can include accuracy, precision, recall, mean Average Precision (mAP), or other task-specific measures. Regularly evaluate the model's performance on the collected video dataset to track progress and identify areas for improvement.
.png)
Conclusion:
Optimising video data collection for ML requires careful planning, diverse content selection, appropriate annotation, and a focus on data quality. By considering key factors such as the ML objective, diversity of content, annotation requirements, data quality, ethical considerations, and evaluation metrics, you can build a robust video dataset that enhances the performance and effectiveness of your ML models. Effective video data collection is a crucial step towards advancing computer vision applications and driving innovation in the field of machine learning.
Comments
Post a Comment