Precision Matters: The Impact of High-Quality Annotation in ADAS ML
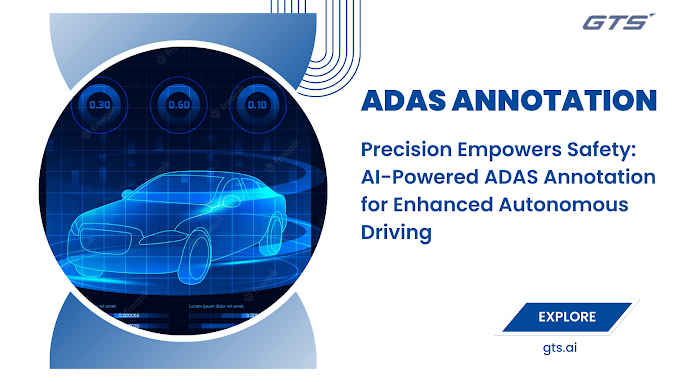
Introduction:
Advanced Driver Assistance Systems (ADAS) are revolutionising the automotive industry by providing intelligent features that enhance vehicle safety and driver experience. Machine learning plays a vital role in powering ADAS, enabling vehicles to perceive and interpret their surroundings. However, the accuracy and reliability of ADAS models heavily depend on high-quality annotation. In this blog post, we will explore the significance of high-quality annotation in ADAS Annotation machine learning and its profound impact on ensuring precision and safety in autonomous driving.
The Role of Annotation in ADAS ML:
Annotation involves labelling and annotating data to provide meaningful information to machine learning algorithms. In ADAS, annotation is used to label objects such as pedestrians, vehicles, traffic signs, and road boundaries in sensor data like images, videos, and LiDAR scans. These annotations serve as ground truth data for training ADAS models, enabling them to recognize and respond to different objects and scenarios accurately.
Accurate Object Detection and Recognition:
High-quality annotation is essential for accurate object detection and recognition in ADAS. Precise labelling of objects in training data helps models learn their characteristics, shapes, and appearances, enabling them to detect and recognize objects in real-world scenarios. Whether it's identifying pedestrians on the road or detecting traffic signs, accurate annotation ensures that ADAS models make informed decisions, enhancing safety and performance.
.png)
Handling Complex Scenarios:
ADAS systems must be capable of handling complex driving scenarios sensor data like Image data collection encountered in real-world environments. High-quality annotation aids in training ADAS models to understand and respond appropriately to challenging situations such as occlusions, adverse weather conditions, and complex road layouts. Properly annotated data enables models to learn the nuances of these scenarios, improving their ability to navigate through complex driving conditions.
Training Robust Perception Models:
ADAS perception models rely on accurate and diverse annotation to develop robust understanding of the environment. Annotated data captured from various viewpoints, lighting conditions, and weather conditions helps models generalise well and perform reliably in different situations. High-quality annotation facilitates the creation of perception models that are resilient to variations, ensuring consistent performance across diverse driving scenarios.
Annotating for Safety-Critical Features:
Certain ADAS features, such as emergency braking or lane-keeping assist, are safety-critical and require precise annotation. Inaccurate or inadequate annotation can lead to false positives or false negatives, compromising the safety of the vehicle and its occupants. High-quality annotation in training data ensures that safety-critical features are accurately identified, reducing the risk of false alarms or missed detections.
Human-in-the-Loop Annotation:
To ensure the highest level of annotation accuracy, human-in-the-loop annotation methodologies are employed. Human annotators, with their expertise and domain knowledge, meticulously label and verify objects in the data. This iterative process involves rigorous quality control measures, continuous feedback, and validation to maintain annotation precision. Human-in-the-loop annotation contributes to the development of accurate and reliable ADAS models.
Continuous Improvement and Adaptation:
ADAS technology is constantly evolving, and annotation plays a crucial role in continuous model improvement. Real-world data collected from ADAS systems can be used to augment and refine the annotation process, allowing models to adapt to new scenarios and edge cases. By continuously updating and improving annotation, ADAS models can stay ahead of emerging challenges, ensuring precision and safety on the road.
Conclusion:
High-quality annotation is paramount in ADAS machine learning to ensure the precision, accuracy, and safety of autonomous driving systems. Proper annotation enables ADAS models to detect and recognize objects, handle complex scenarios, and develop robust perception capabilities. With meticulous human-in-the-loop annotation methodologies and continuous improvement, ADAS annotation sets the foundation for reliable and trustworthy autonomous driving experiences. By prioritising precision in annotation, we pave the way for the future of safe and intelligent autonomous vehicles.
GTS.AI AND ADAS ANNOTATION
Determine if GTS.AI can accommodate specific annotation requirements and customize the annotation process based on your project needs. This may involve defining specific annotation classes, attributes, or requirements. Assess GTS.AI's expertise and experience in ADAS annotation. Consider their track record, client testimonials, or case studies to understand their capabilities and successful projects in this domain
Comments
Post a Comment