Data Collection and Quality Challenges in Deep Learning:
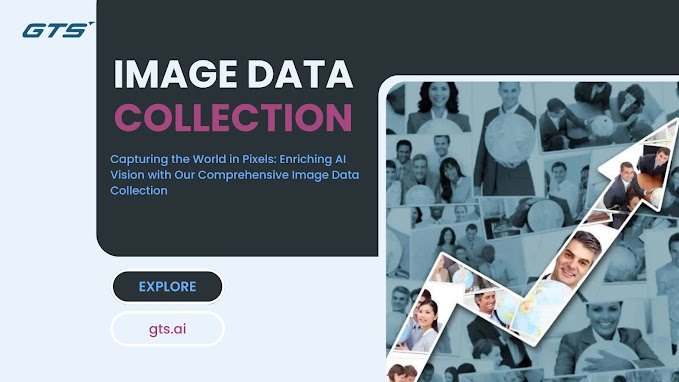.jpg)
Introduction:
In the realm of artificial intelligence (AI) and deep learning, data is the driving force behind the success of cutting-edge algorithms. For image-based applications, such as computer vision and object recognition, high-quality Image Data Collection is a critical aspect of model training. However, the process of gathering, curating, and maintaining image datasets comes with unique challenges that directly impact the performance and reliability of deep learning models. In this blog, we explore the data collection and quality challenges in deep learning from a data-centric AI perspective, focusing on the significance of image data collection for companies seeking to harness the power of AI.
The Power of Image Data in Deep Learning:
Deep learning algorithms excel at image analysis tasks, thanks to their ability to learn intricate patterns and features from large datasets. Image data fuels the training of neural networks, enabling AI models to recognize objects, detect anomalies, and make informed decisions based on visual inputs. The more diverse and high-quality the image dataset, the more accurate and robust the deep learning models become, transforming industries and pushing the boundaries of AI applications.
Data Collection Challenges:
- Annotation Complexity: Annotating image data with precise labels is a time-consuming process, especially for complex objects or densely populated scenes. Expert annotators and sophisticated tools are required to ensure accurate annotations.
- Data Volume: Deep learning models thrive on large-scale datasets. For image-based applications, acquiring a sufficient volume of diverse images and Video Data Collection can be a challenging and resource-intensive task.
- Data Diversity: Image data must encompass a wide variety of objects, backgrounds, lighting conditions, and viewpoints to enhance model generalisation. Ensuring diversity in the dataset requires careful curation and collection from multiple sources.
- Data Privacy: If the image data includes personal or sensitive information, ensuring data privacy and compliance with regulations is vital.
- Data Bias: Biassed image data can lead to biassed model outputs, impacting fairness and inclusivity. Detecting and mitigating bias requires constant vigilance during data collection.
.png)
Data Quality Challenges:
- Noisy Data: Low-quality or noisy images can hinder model training and lead to inaccurate predictions. Cleaning and preprocessing the data is essential to remove noise and artefacts.
- Imbalanced Data: Imbalanced datasets, where certain classes have significantly more samples than others, can skew model performance. Resampling techniques or data augmentation can address this issue.
- Outliers: Outliers or anomalies in the image data may disrupt model training, requiring identification and careful handling.
- Label Accuracy: Inaccurate or inconsistent annotations can mislead the model during training. Regular validation and verification of labels are essential to maintain data quality.
- Data Versioning: As image data evolves, ensuring proper versioning and tracking of changes is crucial for reproducibility and maintaining a reliable data pipeline.
The Role of Image Data Collection Companies:
To overcome the challenges of image data collection and quality, partnering with a professional image data collection company can prove invaluable. These companies offer:
- Expert Annotation: Specialised annotators with domain knowledge ensure accurate and consistent annotations for high-quality image datasets.
- Diverse Data Collection: Image data collection companies can source data from various domains and environments, providing diverse datasets for robust model training.
- Data Privacy Compliance: Reputable data collection companies adhere to strict data privacy guidelines, safeguarding sensitive information in the datasets.
- Data Quality Assurance: Rigorous quality checks and validation processes are performed to ensure data cleanliness and reliability.
.png)
Conclusion:
In the data-centric world of AI and deep learning, image data collection and quality play a pivotal role in shaping the effectiveness of AI models. Addressing the challenges of data collection, diversity, volume, and quality is essential for developing robust and accurate deep learning algorithms. By partnering with a trusted image data collection company, businesses can overcome these challenges and unlock the true potential of AI-powered image applications.
Gts.ai is helpful for image data collection in ml:
Globose Technology Solutions provides the image data set of different documents like driving lisense, identity card, credit card, invoice, receipt, map, menu, newspaper, passport, etc. Our services scope covers a wide area of Image Data Collection and image data annotation services for all forms of machine learning and deep learning applications. As part of our vision to become one of the best deep learning image data collection centers globally, GTS is on the move to providing the best image data collection and classification dataset that will make every computer vision project a huge success. Our Data Collection Company are focused on creating the best image database regardless of your AI model.
Comments
Post a Comment