Human-Annotated vs. Self-Annotated ADAS Data: A Comparative Analysis
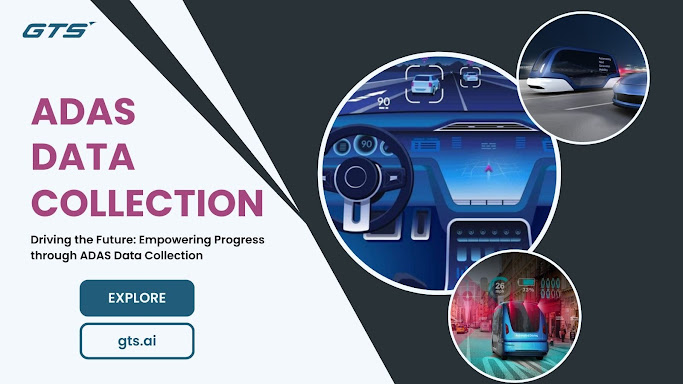
Introduction:
As the automotive industry continues to embrace the revolution of Advanced Driver Assistance Systems (ADAS), data collection plays a pivotal role in the development and deployment of these cutting-edge technologies. Accurate and comprehensive data is essential to ensure the safety and reliability of ADAS functionalities. However, there are two primary methods of data annotation - human-annotated and self-annotated. In this blog, we will delve into the world of ADAS Data Collection and conduct a comparative analysis of human-annotated versus self-annotated data to understand their respective advantages and limitations.
Understanding ADAS Data Collection:
ADAS technology has seen remarkable growth over the years, promising enhanced safety and convenience for drivers. Sensors, cameras, and radar systems in modern vehicles collect a vast amount of data from the surrounding environment. This data includes information about road conditions, nearby vehicles, pedestrians, and various other objects. To make sense of this data and train ADAS algorithms effectively, it needs to be accurately annotated.
The Role of Data Annotation:
Data annotation is the process of labelling the collected raw data to provide it with context and meaning. It involves marking objects of interest and their characteristics in the data, making it understandable for machine learning algorithms. Properly annotated data is crucial for training ADAS systems to recognize and respond appropriately to different scenarios on the road.
Human-Annotated ADAS Data:
Human annotation involves the manual labelling of data by trained experts. These human annotators meticulously identify and label objects and their attributes in the collected data. This method offers several advantages:
- Precision and Accuracy: Human annotators bring their expertise, ensuring accurate and detailed annotations, which are vital for training high-performing ADAS Annotation algorithms.
- Handling Complexity: Complex scenarios on the road can be accurately annotated by humans, enabling ADAS systems to handle challenging situations effectively.
- Adaptability: Human annotators can quickly adapt to changing requirements and update annotations accordingly, providing flexibility during the data collection process.

Limitations of Human-Annotated Data:
While human annotation is a reliable method, it does have some limitations:
- Time-Consuming: Human annotation can be time-consuming, especially when dealing with large datasets, potentially leading to higher costs and longer development cycles.
- Subjectivity: Human annotation may involve some degree of subjectivity, as different annotators may interpret certain scenarios differently.
Self-Annotated ADAS Data:
Self-annotation, on the other hand, leverages the power of artificial intelligence and machine learning algorithms to automatically label data. Some benefits of self-annotation include:
- Speed and Scalability: Self-annotation is faster and more scalable, enabling the processing of large volumes of data in a shorter time.
- Consistency: Machine learning algorithms can provide consistent annotations, reducing the risk of human errors and discrepancies.
- Cost-Effective: By automating the annotation process, self-annotation can potentially lower overall data collection costs.
Limitations of Self-Annotated Data:
While self-annotation is efficient, it does have certain limitations:
- Reliability: The accuracy of self-annotation heavily relies on the quality of the machine learning models used, which may not be as robust as human annotators in complex scenarios.
- Unforeseen Scenarios: Self-annotation may struggle to handle situations that deviate significantly from the data it was initially trained on.
Achieving Optimal Results: Combining Human and Self-Annotation
To address the limitations of both methods and achieve the best results, a hybrid approach can be adopted. By combining human-annotated data to handle complex scenarios and self-annotated data for faster processing and scalability, automotive companies can create comprehensive datasets for ADAS training.
.png)
Conclusion:
In conclusion, ADAS data collection is a critical aspect of developing safer and more reliable autonomous driving technologies. Both human-annotated and self-annotated data have their advantages and limitations. While human annotation offers precision and adaptability, it can be time-consuming and subjective. On the other hand, self-annotation is fast and scalable, but may lack the reliability to handle complex scenarios. For the most effective ADAS data collection, a hybrid approach that combines the strengths of both methods is recommended. By doing so, automotive companies can ensure that their ADAS systems are thoroughly trained and capable of meeting the challenges of tomorrow's roads, ultimately advancing the safety and convenience of driving for all.
Comments
Post a Comment