The Dos and Don'ts of Image Data Collection in Machine Learning
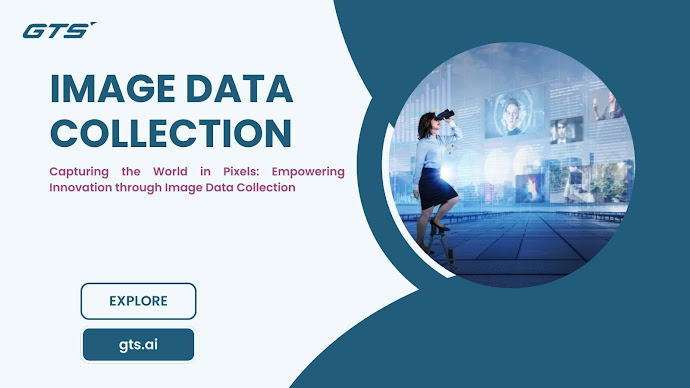
Introduction:
In the world of machine learning, Image Data Collection forms the bedrock upon which powerful AI image models are built. The process of gathering and preparing image data sets the stage for the accuracy and effectiveness of image recognition, object detection, and other computer vision applications. However, successful image data collection requires careful consideration and adherence to certain best practices. In this blog, we will explore the dos and don'ts of image data collection in machine learning, enabling you to create robust and reliable AI models.
The Dos of Image Data Collection:
- Define Clear Objectives: Before diving into data collection, it's crucial to establish clear objectives for your machine learning project. Understand what you want the AI model to accomplish and the specific categories or objects it should recognize. This clarity will guide your data collection efforts in the right direction.
- Diversify Your Data: Aim to collect a diverse range of images that cover different variations of objects, angles, lighting conditions, and backgrounds. Diverse data ensures that your AI model can generalise well to real-world scenarios, avoiding overfitting on specific data patterns.
- Maintain Quality Standards: Emphasise quality over quantity when collecting images. Blurry, poorly lit, or low-resolution images can hinder the model's performance. Verify and filter images during the collection process to maintain high-quality data.
- Ensure Data Consistency: Consistency in data collection is vital for training accurate AI models. Maintain uniformity in image sizes, formats, and annotation standards to streamline the training process and avoid unnecessary complications.
- Label Data Accurately: Accurate and precise annotations are the foundation of supervised learning in image data. Invest time and effort in meticulous labelling to ensure your model learns from correctly annotated data.
- Use Data Augmentation: Data augmentation techniques, such as rotation, flipping, and scaling, can significantly increase the diversity of your dataset without the need for extensive manual collection. This technique enhances the model's ability to handle different variations of images.
.png)
The Don'ts of Image Data Collection:
Avoid Biassed Data: Biassed data can lead to discriminatory and unfair AI models. Be cautious about selecting images that disproportionately represent certain demographics or exclude others, as it may result in biassed predictions.
Steer Clear of Copyrighted Content: Using copyrighted images and Video Data Collection without proper authorization can lead to legal issues. Respect intellectual property rights and opt for publicly available or licensed images for your dataset.
Don't Overlook Data Privacy: If your image and Vdataset contains sensitive information or personally identifiable details, ensure compliance with data privacy regulations. Anonymize or obtain proper consent before using such data.
Refrain from Insufficient Data: Insufficient data can hinder the model's ability to learn effectively. Avoid under-represented categories and strive to balance the dataset to achieve optimal performance.
Avoid Low-Quality Data Sources: Relying on unreliable or unverified sources can introduce noise and inaccuracies in your dataset. Verify the credibility of data sources and prioritise reputable repositories.
.png)
Conclusion:
Image data collection is a fundamental step in creating powerful and reliable AI image models. Following the dos and don'ts outlined in this blog will ensure that your dataset is robust, diverse, and representative of real-world scenarios. Clear objectives, high-quality data, accurate annotations, and ethical considerations are the pillars that support the success of your machine learning project. By diligently collecting and preparing image data, you set the stage for training AI models that can make accurate predictions, facilitate advanced computer vision applications, and contribute to transformative advancements across various industries.
Comments
Post a Comment