Achieving Precision and Safety: Optimising ADAS Data Collection for Effective ML Models
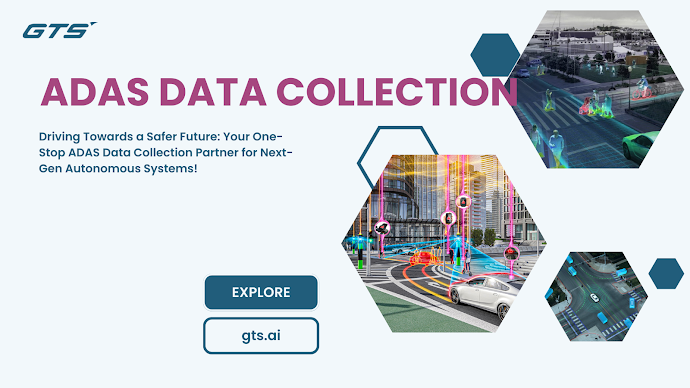
Introduction:
Advanced Driver Assistance Systems (ADAS) have revolutionised the automotive industry by enhancing vehicle safety and providing an improved driving experience. ADAS relies heavily on machine learning (ML) models to analyse real-time data and make intelligent decisions. However, the effectiveness of these models largely depends on the quality and accuracy of the data they are trained on. This is where ADAS Data Collection plays a crucial role. In this blog post, we will explore the significance of ADAS data collection, its challenges, and strategies to optimise it for achieving precision and safety in ML models.
Importance of ADAS Data Collection:
ADAS systems require vast amounts of diverse and representative data to learn and recognize various road scenarios, objects, and potential hazards. Data collection serves as the foundation for building robust ML models that can accurately identify and respond to different driving situations. By collecting and analysing real-world data, automotive companies can fine-tune their ADAS algorithms, resulting in safer and more reliable autonomous vehicles.
Challenges in ADAS Data Collection:
- Data Volume: Collecting a large volume of high-quality data that covers a wide range of driving scenarios can be a significant challenge. Gathering data from various environments, weather conditions, and traffic patterns is essential to train ML models effectively.
- Data Diversity: ADAS systems need to be trained on diverse data to handle various road conditions, driving styles, and potential obstacles. Ensuring a representative dataset that encompasses different geographic locations, traffic densities, and driving cultures is crucial for reliable ML models.
- Data Annotation: Annotating collected data with accurate labels and annotations is a time-consuming and labour-intensive task. Human involvement is often necessary to label objects, road markings, and other relevant information in the data, adding complexity to the data collection process.

Optimising ADAS Data Collection:
- Collaborative Data Sharing: Collaboration between automotive manufacturers, researchers, and regulatory bodies can help establish data-sharing frameworks. Sharing anonymized and standardised datasets can benefit the entire industry by increasing the diversity and size of available training data.
- Sensor Fusion: Integrating multiple sensors, such as cameras, lidars, radars, and GPS, enables comprehensive data collection from different perspectives. Sensor fusion allows for a more accurate perception of the environment, resulting in better ML model training.
- Data Augmentation: To supplement the collected data, techniques such as data augmentation can be employed. By applying transformations like image rotation, scaling, and noise addition, the dataset can be expanded, increasing its diversity and generalizability.
- Active Learning: Implementing active learning techniques can make the data collection process more efficient. By using ML models to identify uncertain or challenging examples, human annotators can focus their efforts on labelling the most informative data points, optimising the annotation process.
- Continuous Data Collection: ADAS Annotation systems need to adapt and improve over time. Implementing continuous data collection practices through Over-The-Air (OTA) updates ensures that ML models can be regularly retrained with fresh and relevant data, reflecting real-world changes and improvements.
.gif)
Conclusion:
ADAS data collection is a critical component in building effective ML models that power advanced driver assistance systems. By addressing challenges such as data volume, diversity, and annotation, and leveraging strategies like collaborative data sharing, sensor fusion, data augmentation, active learning, and continuous data collection, automotive companies can optimise their ADAS data collection processes. This optimization leads to ML models that are more precise, reliable, and capable of ensuring safety on the roads. As the automotive industry progresses towards full autonomy, prioritising ADAS data collection will play a pivotal role in achieving the ultimate goal of safe and efficient self-driving vehicles.
How GTS.AI Can Help You?
As vehicles evolve into intelligent entities, AI ADAS data collection emerges as a defining factor. Globose Technology Solutions Pvt Ltd (GTS) is at the forefront of this evolution, providing AI models with the data needed to make informed decisions, enhance safety, and revolutionize the driving experience. As we envision a future of autonomous mobility and connected vehicles, GTS's role in onboard intelligence through AI ADAS data collection remains an essential component of this transformative journey.
Comments
Post a Comment