Incorporating Domain Knowledge in Text Data Collection for Machine Learning
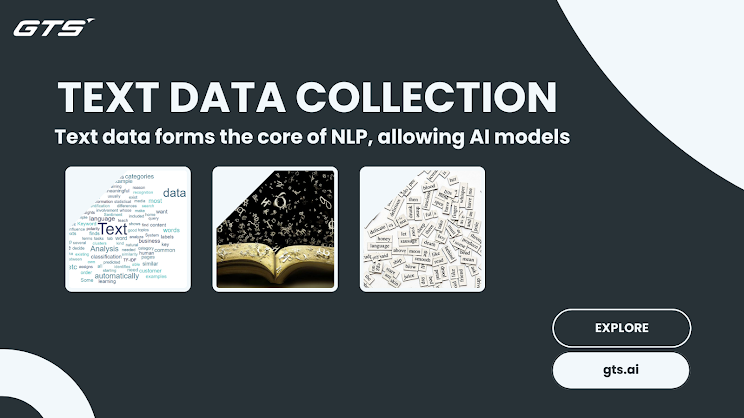
Introduction:
The success of machine learning models heavily relies on the quality and relevance of the training data they are exposed to. When it comes to text data, incorporating domain knowledge during the collection process is crucial for building effective models in specific industries or areas of expertise. By gathering text data that is tailored to a particular domain, machine learning algorithms can better understand the intricacies, context, and nuances of the subject matter. In this blog post, we will explore the importance of incorporating domain knowledge in Text Data Collection for machine learning and its impact on model performance.
Understanding the Domain:
Domain knowledge refers to the expertise, understanding, and familiarity with a specific subject area or industry. By leveraging domain knowledge during text data collection, we gain insights into the specific language, terminology, and concepts used within that domain. This understanding helps curate a more relevant and accurate dataset, enabling the machine learning model to capture the unique characteristics of the domain and improve its performance.
Identifying Relevant Sources:
Domain knowledge plays a crucial role in identifying relevant sources of text data. Understanding the key publications, websites, journals, forums, or social media platforms frequented by domain experts or practitioners can help pinpoint valuable textual resources. By focusing on authoritative and reliable sources within the domain, we ensure that the collected data reflects the latest trends, developments, and discussions relevant to the target industry.
.png)
Designing Effective Queries and Keywords:
Applying domain knowledge to the design of queries and keywords is essential for targeted text data collection. By using domain-specific terms, jargon, and phrases in search queries, we can retrieve more relevant and specific Text-to-Speech Datasets content. This approach helps filter out noise and irrelevant information, ensuring that the collected dataset is focused on the specific domain of interest. Domain experts can provide valuable guidance in formulating effective search queries that capture the nuances and intricacies of the subject matter.
Annotating and Labelling the Data:
Domain knowledge is instrumental in annotating and labelling the collected text data. Domain experts can contribute their expertise in tagging, categorising, or labelling the textual content based on the specific requirements of the machine learning task. Their understanding of the subject matter allows for more accurate and meaningful annotations, which in turn enhances the model's ability to learn and make informed predictions within the domain.
Handling Domain-specific Challenges:
Different domains present unique challenges in text data collection. For example, in medical or legal domains, ensuring data privacy and confidentiality is crucial. Domain knowledge helps navigate these challenges by understanding the legal and ethical considerations, obtaining proper permissions, and anonymizing sensitive information appropriately. By incorporating domain expertise, we can overcome domain-specific hurdles and collect high-quality data that meets legal and ethical standards.
Iterative Refinement and Feedback:
Domain knowledge serves as a continuous feedback loop during the text data collection process. As the model is trained and evaluated, domain experts provide valuable insights and feedback on the model's performance within the domain. This feedback helps identify areas for improvement, fine-tune the collection process, and refine the dataset. The iterative nature of incorporating domain knowledge ensures that the machine learning model aligns with the evolving needs and intricacies of the target domain.
.gif)
Conclusion:
Incorporating domain knowledge in text data collection is essential for building effective machine learning models in specific industries or areas of expertise. By understanding the nuances, terminologies, and context within a domain, we can curate a relevant and accurate dataset that empowers the model to learn and make informed predictions. Domain expertise guides the identification of relevant sources, formulation of effective queries, annotation of data, handling domain-specific challenges, and continuous refinement of the model. By integrating domain knowledge into text data collection, we pave the way for powerful and industry-specific machine learning applications that drive innovation and solve complex problems within a given domain.
Comments
Post a Comment