The Human Touch in AI: The Critical Role of Data Annotation
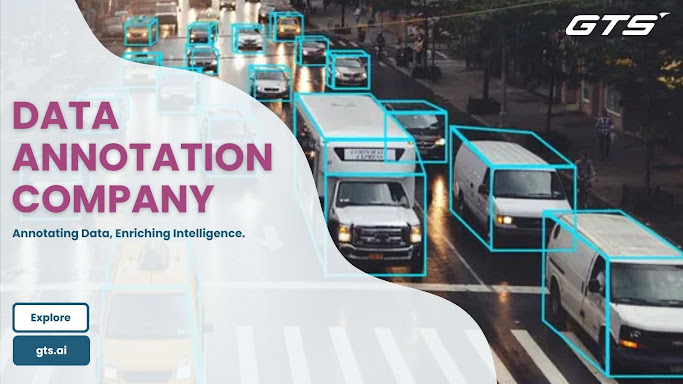
Introduction:
Data Annotation is a critical process in the field of machine learning and artificial intelligence (AI), serving as the foundation upon which models learn, understand, and interpret the world around them. This blog post aims to explore the nuances of data annotation, its importance, the different types of annotations, challenges, and best practices for effective data annotation.
Understanding Data Annotation
Data annotation is the process of labeling or tagging data in various formats, such as text, images, and videos, to make it understandable for machine learning models. This labeling can include identifying objects in images, annotating sentiment in text, or categorizing audio files. The quality and accuracy of data annotation directly influence the performance of AI models, making it a pivotal step in the development of intelligent systems.
Importance of Data Annotation
The significance of data annotation cannot be overstated. It enables machine learning models to accurately interpret the data they are fed, learning from examples to make predictions or decisions based on new, unseen data. Without properly annotated data, models could misinterpret inputs, leading to inaccurate outcomes or biased decisions. Therefore, data annotation is crucial for:
- Training Machine Learning Models: Annotated data is used to train models on how to process and analyze data correctly.
- Improving Model Accuracy: High-quality annotation ensures that models learn the right patterns and nuances.
- Enabling AI Applications: From autonomous vehicles and facial recognition to natural language processing and predictive analytics, data annotation empowers a wide range of AI applications.
Types of Data Annotation
Data annotation encompasses various forms, each tailored to specific types of data and applications:
- Image and Video Annotation: This includes object detection, bounding boxes, segmentation, and landmark annotation to help models recognize and interpret visual data.
- Text Annotation: Natural language processing (NLP) relies on text annotation, which involves sentiment analysis, entity recognition, and categorization to understand and generate human language.
- Audio Annotation: Transcribing, labeling emotions, and identifying key sounds within audio clips are essential for developing voice-activated assistants, speech recognition systems, and other audio-based applications.

Challenges in Data Annotation
Despite its importance, data annotation is not without its challenges:
- Scale: Annotating data can be time-consuming and expensive, especially for large datasets required by deep learning models.
- Quality Control: Ensuring the consistency and accuracy of annotations across large datasets is challenging but crucial for model performance.
- Bias: Unintentional bias in annotated data can lead to biased AI models, making fairness and representation key considerations in the annotation process.
Best Practices for Effective Data Annotation
To overcome these challenges and ensure the effectiveness of data annotation, several best practices can be followed:
- Define Clear Annotation Guidelines: Clear, detailed guidelines ensure consistency and accuracy in annotations.
- Use the Right Tools: Leveraging annotation tools can streamline the process, improve efficiency, and enhance accuracy.
- Quality Assurance: Implementing multiple rounds of checks and balances, including automated and manual reviews, can help maintain high annotation quality.
- Diverse Annotation Teams: A diverse team of annotators can help mitigate bias in the data, leading to more robust and fair AI models.
- Continuous Improvement: Feedback loops between annotators and model developers can help refine annotation guidelines and improve model performance over time.
Conclusion
Data annotation is a foundational element in the development of accurate and fair machine learning models. By understanding its importance, types, challenges, and best practices, stakeholders in the AI field can better navigate the complexities of creating intelligent systems. As AI continues to evolve, the role of data annotation in ensuring these systems understand and interact with the world accurately and ethically will only grow in importance.
Data Annotation Services With GTS Experts
Globose Technology Solutions stands as a pivotal player in the realm of data annotation services, providing essential tools and expertise that significantly enhance the quality and efficiency of AI model training. Their sophisticated AI-driven solutions streamline the annotation process, ensuring accuracy, consistency, and speed.
Comments
Post a Comment