Data Annotation Services: The Backbone of AI and Machine Learning
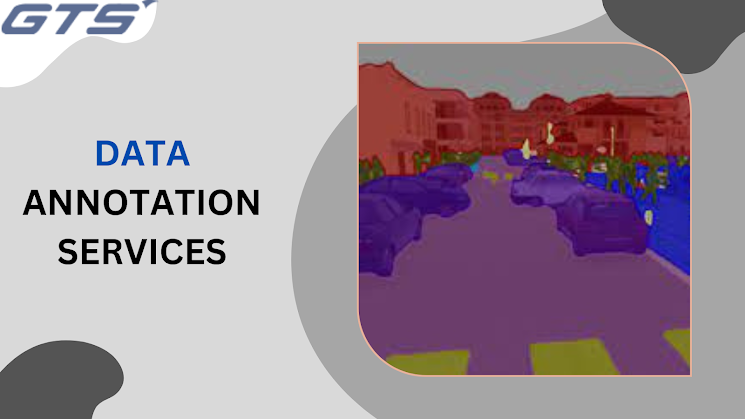
Introduction:
In the ever-evolving world of artificial intelligence (AI) and machine learning (ML), Data Annotation Services have emerged as a critical backbone, enabling machines to understand and interpret the world around them. As we venture deeper into the realms of automation, natural language processing, and computer vision, the significance of high-quality data annotation becomes increasingly apparent.
What is Data Annotation?
Data annotation is the process of labeling or tagging data in various formats, such as text, images, videos, and audio, to make it understandable for machine learning algorithms. These annotations can range from simple tags to complex, multi-layered labels, depending on the requirements of the AI application. For instance, in image annotation, objects within an image may be outlined and labeled to help a computer vision model recognize and classify similar objects in new images.
The Role of Data Annotation Services in AI and ML
The accuracy and effectiveness of AI and ML models are heavily dependent on the quality of the training data. Annotated data serves as the training material that teaches these models to recognize patterns, make predictions, and perform tasks. Without proper annotation, models can struggle to interpret data correctly, leading to errors and inefficiencies.
Data annotation services provide the expertise and resources needed to handle large volumes of data, ensuring that it is accurately and consistently annotated. These services often employ teams of annotators who are trained to label data according to specific guidelines, ensuring that the annotations are of high quality and suitable for training robust AI models.
Types of Data Annotation
Data annotation encompasses a wide range of techniques, each suited to different types of data and applications:
- Image and Video Annotation: This involves labeling visual content with tags, bounding boxes, polygons, or keypoints to identify objects, actions, or features. It is crucial for applications like autonomous vehicles, facial recognition, and medical imaging.
- Text Annotation: Text data is annotated to identify entities, sentiments, or relationships, which is essential for natural language processing tasks such as sentiment analysis, chatbots, and language translation.
- Audio Annotation: In audio annotation, sound clips are labeled to identify specific sounds, words, or emotions. This is important for voice assistants, speech recognition systems, and audio analysis applications.
- Semantic Annotation: This involves adding metadata to data to provide context and meaning, enabling machines to understand the semantics of the data. It is used in various applications, including web search, content recommendation, and knowledge graphs.

Challenges in Data Annotation
Despite its importance, data annotation is not without its challenges. It can be a time-consuming and labor-intensive process, especially for large datasets. Ensuring consistency and accuracy across annotations is also a significant challenge, as it requires clear guidelines and quality control measures. Additionally, annotating data for complex or niche applications may require specialized knowledge, making it difficult to find qualified annotators.
The Future of Data Annotation Services
As AI and ML continue to advance, the demand for high-quality annotated data is only set to increase. Data annotation services are evolving to meet this demand, leveraging technology to improve efficiency and accuracy. Automation and semi-automated annotation tools are becoming more common, reducing the manual effort required and speeding up the annotation process. Machine learning models are also being used to pre-annotate data, which annotators can then review and refine.
Furthermore, the rise of crowd-sourcing platforms has made it easier to access a diverse pool of annotators, enabling more scalable and flexible annotation services. These platforms also allow for the democratization of data annotation, giving more people the opportunity to contribute to the development of AI and ML technologies.
Conclusion
Data annotation services are an indispensable part of the AI and ML ecosystem, providing the foundation upon which intelligent systems are built. As technology continues to evolve, the importance of high-quality, accurately annotated data will only grow. By embracing innovation and leveraging the power of the crowd, data annotation services are poised to meet the challenges of the future, enabling the continued advancement of AI and machine learning.
Comments
Post a Comment