Unlocking the Potential of OCR: Insights into Effective Data Collection
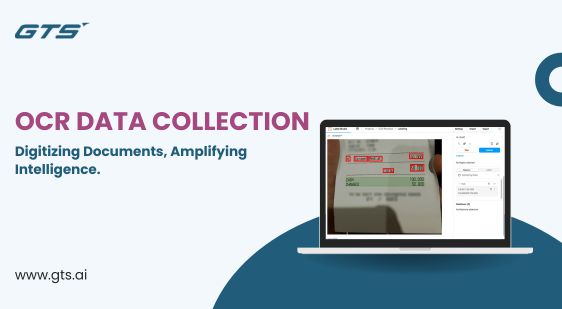
Introduction:
Optical Character Recognition (OCR) technology has revolutionized the way businesses and organizations process vast amounts of data. By converting different types of documents into machine-readable text, OCR opens up new opportunities for automation, data analysis, and information retrieval. However, the effectiveness of OCR technology largely depends on the quality and variety of the datasets used to train these systems. In this blog, we delve deep into the intricacies of OCR Data Collection, exploring the types of data needed, challenges faced, and best practices for assembling a robust OCR dataset.
Understanding OCR and Its Importance
OCR technology uses machine learning models to decode text from images and scanned documents. This ability transforms static images into dynamic data that can be edited, searched, and managed. The applications of OCR are diverse and impactful, ranging from digitizing historical documents and automating data entry to enhancing accessibility for the visually impaired by converting written content into speech.
Types of Data Required for OCR
The foundation of a competent OCR system lies in its training dataset. The data collected must be as diverse as the applications it serves. Here are some key types of data essential for training effective OCR systems:
- Printed Text Data: Includes books, newspapers, magazines, and printed documents. This category is crucial for training basic OCR models that recognize standard fonts and layouts.
- Handwritten Text Data: Consists of handwritten notes, forms, and letters. This data helps in training more sophisticated OCR models that can understand varied handwriting styles.
- Multilingual Text Data: Datasets comprising multiple languages, including those with non-Latin scripts such as Arabic, Chinese, or Hindi. Multilingual data is vital for creating OCR systems that can be deployed globally.
- Noisy Background Data: Images with text over complex backgrounds or under poor lighting conditions. Training models with such data ensures robustness in real-world scenarios.
- Annotated Text Data: Beyond raw images, annotated data with labeled Text to Speech Dataset positions and character segmentation is crucial for training accurate OCR models.
Challenges in OCR Data Collection
Collecting a comprehensive and effective dataset for OCR poses several challenges:
- Variability and Diversity: Ensuring the dataset covers a wide range of fonts, styles, layouts, and languages is essential but challenging. The more diverse the data, the more capable the OCR system will be.
- Quality of Images: Poor image quality can significantly degrade OCR accuracy. Factors such as resolution, lighting, and noise play critical roles in the usability of the data.
- Legal and Ethical Considerations: Many documents contain sensitive information. Adhering to data protection regulations and ethical guidelines is paramount in OCR data collection.
- Annotation Efforts: The process of annotating text within images is labor-intensive and requires a high level of accuracy to ensure the model trains correctly.
Best Practices for Effective OCR Data Collection
To overcome these challenges and build a potent OCR dataset, follow these best practices:
- Diverse Source Acquisition: Collect data from a variety of sources to ensure a rich mix of text types, styles, and backgrounds.
- High-Quality Scanning Procedures: Use high-quality scanners and capture settings to maintain the integrity of the original text.
- Robust Annotation Protocols: Develop detailed annotation guidelines to ensure consistency and accuracy across the dataset.
- Ethical Data Practices: Obtain all necessary permissions for data usage and ensure compliance with data protection laws.
- Continuous Dataset Evaluation and Augmentation: Regularly evaluate the performance of your OCR models with real-world tests and continuously augment the dataset with new data that addresses any identified gaps.
Conclusion
The effectiveness of OCR systems hinges on the quality of the data used in their development. By understanding the types of data required, recognizing the challenges in collecting such data, and implementing best practices, organizations can greatly enhance their OCR capabilities. As technology evolves, the scope of OCR applications will expand, further underscoring the importance of robust and diverse datasets. By investing in comprehensive OCR data collection, businesses can unlock new efficiencies and insights, driving innovation and competitive advantage.
At Globose Technology Solutions, we are committed to providing state-of-the-art OCR solutions to meet the specific needs of our customers. Contact us today to learn more about how OCR can transform your data collection workflow..
Comments
Post a Comment